Synthetic intelligence algorithms are more and more being utilized in monetary companies — however they arrive with some critical dangers round discrimination.
Sadik Demiroz | Photodisc | Getty Pictures
AMSTERDAM — Synthetic intelligence has a racial bias downside.
From biometric identification techniques that disproportionately misidentify the faces of Black folks and minorities, to purposes of voice recognition software program that fail to differentiate voices with distinct regional accents, AI has so much to work on on the subject of discrimination.
And the issue of amplifying present biases may be much more extreme on the subject of banking and monetary companies.
Deloitte notes that AI techniques are finally solely nearly as good as the info they’re given: Incomplete or unrepresentative datasets may restrict AI’s objectivity, whereas biases in growth groups that prepare such techniques may perpetuate that cycle of bias.
A.I. may be dumb
Nabil Manji, head of crypto and Web3 at Worldpay by FIS, stated a key factor to know about AI merchandise is that the power of the know-how relies upon so much on the supply materials used to coach it.
“The factor about how good an AI product is, there’s form of two variables,” Manji informed CNBC in an interview. “One is the info it has entry to, and second is how good the big language mannequin is. That is why the info aspect, you see corporations like Reddit and others, they’ve come out publicly and stated we’re not going to permit corporations to scrape our knowledge, you are going to need to pay us for that.”
As for monetary companies, Manji stated loads of the backend knowledge techniques are fragmented in several languages and codecs.
“None of it’s consolidated or harmonized,” he added. “That’s going to trigger AI-driven merchandise to be so much much less efficient in monetary companies than it is likely to be in different verticals or different corporations the place they’ve uniformity and extra fashionable techniques or entry to knowledge.”

Manji advised that blockchain, or distributed ledger know-how, may function a option to get a clearer view of the disparate knowledge tucked away within the cluttered techniques of conventional banks.
Nonetheless, he added that banks — being the closely regulated, slow-moving establishments that they’re — are unlikely to maneuver with the identical pace as their extra nimble tech counterparts in adopting new AI instruments.
“You have acquired Microsoft and Google, who like during the last decade or two have been seen as driving innovation. They cannot sustain with that pace. After which you consider monetary companies. Banks aren’t recognized for being quick,” Manji stated.
Banking’s A.I. downside
Rumman Chowdhury, Twitter’s former head of machine studying ethics, transparency and accountability, stated that lending is a primary instance of how an AI system’s bias towards marginalized communities can rear its head.
“Algorithmic discrimination is definitely very tangible in lending,” Chowdhury stated on a panel at Money20/20 in Amsterdam. “Chicago had a historical past of actually denying these [loans] to primarily Black neighborhoods.”
Within the Thirties, Chicago was recognized for the discriminatory observe of “redlining,” wherein the creditworthiness of properties was closely decided by the racial demographics of a given neighborhood.
“There could be a large map on the wall of all of the districts in Chicago, and they might draw crimson traces via the entire districts that had been primarily African American, and never give them loans,” she added.
“Quick ahead just a few a long time later, and you’re creating algorithms to find out the riskiness of various districts and people. And when you might not embody the info level of somebody’s race, it’s implicitly picked up.”
Certainly, Angle Bush, founding father of Black Girls in Synthetic Intelligence, a company aiming to empower Black ladies within the AI sector, tells CNBC that when AI techniques are particularly used for mortgage approval choices, she has discovered that there’s a threat of replicating present biases current in historic knowledge used to coach the algorithms.
“This can lead to automated mortgage denials for people from marginalized communities, reinforcing racial or gender disparities,” Bush added.
“It’s essential for banks to acknowledge that implementing AI as an answer might inadvertently perpetuate discrimination,” she stated.
Frost Li, a developer who has been working in AI and machine studying for over a decade, informed CNBC that the “personalization” dimension of AI integration can be problematic.
“What’s fascinating in AI is how we choose the ‘core options’ for coaching,” stated Li, who based and runs Loup, an organization that helps on-line retailers combine AI into their platforms. “Typically, we choose options unrelated to the outcomes we wish to predict.”
When AI is utilized to banking, Li says, it is tougher to establish the “wrongdoer” in biases when the whole lot is convoluted within the calculation.
“A great instance is what number of fintech startups are particularly for foreigners, as a result of a Tokyo College graduate will not be capable to get any bank cards even when he works at Google; but an individual can simply get one from group school credit score union as a result of bankers know the native colleges higher,” Li added.
Generative AI isn’t normally used for creating credit score scores or within the risk-scoring of customers.
“That isn’t what the software was constructed for,” stated Niklas Guske, chief working officer at Taktile, a startup that helps fintechs automate decision-making.
As a substitute, Guske stated probably the most highly effective purposes are in pre-processing unstructured knowledge akin to textual content recordsdata — like classifying transactions.
“These alerts can then be fed right into a extra conventional underwriting mannequin,” stated Guske. “Subsequently, Generative AI will enhance the underlying knowledge high quality for such choices relatively than change widespread scoring processes.”
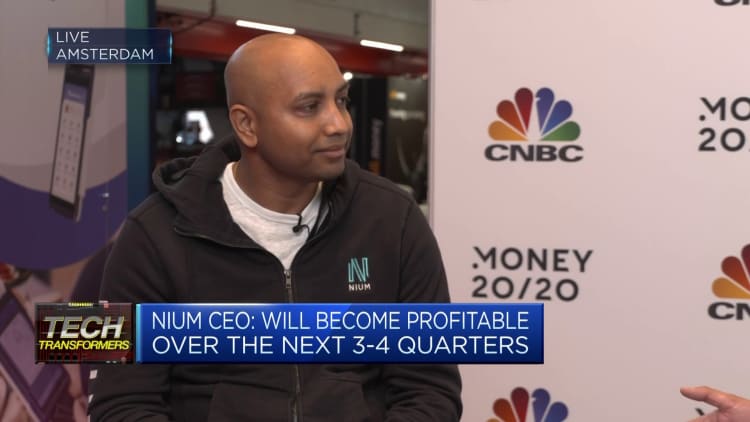
However it’s additionally tough to show. Apple and Goldman Sachs, for instance, had been accused of giving ladies decrease limits for the Apple Card. However these claims had been dismissed by the New York Division of Monetary Providers after the regulator discovered no proof of discrimination primarily based on intercourse.
The issue, based on Kim Smouter, director of anti-racism group European Community Towards Racism, is that it may be difficult to substantiate whether or not AI-based discrimination has truly taken place.
“One of many difficulties within the mass deployment of AI,” he stated, “is the opacity in how these choices come about and what redress mechanisms exist had been a racialized particular person to even discover that there’s discrimination.”
“People have little information of how AI techniques work and that their particular person case might, the truth is, be the tip of a systems-wide iceberg. Accordingly, it is also tough to detect particular situations the place issues have gone improper,” he added.
Smouter cited the instance of the Dutch youngster welfare scandal, wherein 1000’s of profit claims had been wrongfully accused of being fraudulent. The Dutch authorities was pressured to resign after a 2020 report discovered that victims had been “handled with an institutional bias.”
This, Smouter stated, “demonstrates how rapidly such disfunctions can unfold and the way tough it’s to show them and get redress as soon as they’re found and within the meantime vital, usually irreversible harm is completed.”
Policing A.I.’s biases
Chowdhury says there’s a want for a world regulatory physique, just like the United Nations, to handle a few of the dangers surrounding AI.
Although AI has confirmed to be an progressive software, some technologists and ethicists have expressed doubts concerning the know-how’s ethical and moral soundness. Among the many high worries business insiders expressed are misinformation; racial and gender bias embedded in AI algorithms; and “hallucinations” generated by ChatGPT-like instruments.
“I fear fairly a bit that, as a consequence of generative AI, we’re getting into this post-truth world the place nothing we see on-line is reliable — not any of the textual content, not any of the video, not any of the audio, however then how can we get our info? And the way can we make sure that info has a excessive quantity of integrity?” Chowdhury stated.
Now could be the time for significant regulation of AI to come back into drive — however figuring out the period of time it would take regulatory proposals just like the European Union’s AI Act to take impact, some are involved this may not occur quick sufficient.
“We name upon extra transparency and accountability of algorithms and the way they function and a layman’s declaration that enables people who aren’t AI consultants to guage for themselves, proof of testing and publication of outcomes, unbiased complaints course of, periodic audits and reporting, involvement of racialized communities when tech is being designed and regarded for deployment,” Smouter stated.
The AI Act, the primary regulatory framework of its type, has integrated a elementary rights method and ideas like redress, based on Smouter, including that the regulation will likely be enforced in roughly two years.
“It might be nice if this era may be shortened to ensure transparency and accountability are within the core of innovation,” he stated.
